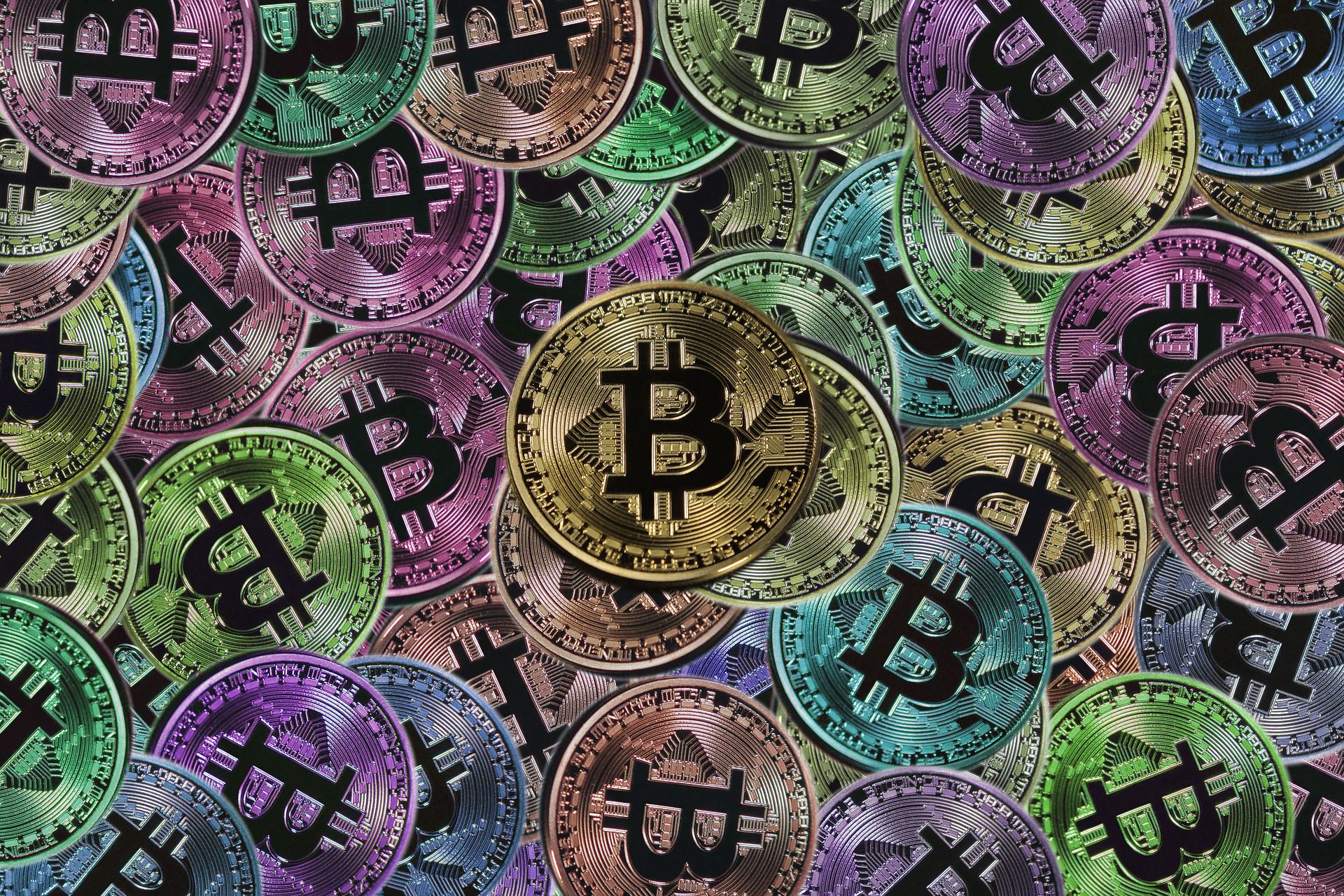
Backpage and Bitcoin: Uncovering Human Traffickers
Sex trafficking and slavery remain amongst the most grievous issues the world faces, supporting a multi-billion dollar industry that cuts across all nationalities and people groups [13]. With the advent of the Internet, many new avenues have opened up to support this pernicious business, including sites for online classified ads selling sex [11]. Although these ad sites provide a significant source of potentially incriminating data for law enforcement, monitoring these sites is unfortunately a labor-intensive task. The rate of new ads per day can reach into the thousands, depending on the website [14]. In addition, the nature of the advertising content can have a uniquely damaging psychological toll on its viewers. Picking out signs of trafficking requires domain expertise, creating an additional barrier for analytics. This problem space is made all the more difficult by the dearth of ground truth, e.g. ads known to be tied to trafficking activity vs. other consensual activity.
In conversation with our NGO and law enforcement collaborators, we have found that there is a real need for tools able to group ads by true owner. Such a tool would allow officers to confidently use timing and location information to distinguish between ads posted by women voluntarily in this industry vs. those by women and children forcibly trafficked. For example, groups of ads—posted by the same owner—that advertise multiple different women across multiple different states at a high ad output rate, is a strong indicator of trafficking. In this case, our goal is to distinguish which ads are owned by the same person or persons. This information can then be used to nd traffickers, connections between pimps, or even trafficking networks.
All of the existing work in this problem space to date uses hard identifiers like phone numbers and email address links to define ownership. This is known to be unreliable (as criminal organizations regularly change their phone numbers/use burner phones, and the cost of creating a new email address is low) but is the best link currently available. In fact, most of the work in this domain has focused on understanding the online environment that supports this industry through surveys and manual analysis ([2, 11, 14]). Almost no work has been done in building tools that can automatically process and classify these ads [4].
The aim of this paper is to develop and demonstrate automatic techniques for clustering sex ads by owner 1 . We designed two such techniques. The first is a machine learning stylometry classifier that determines whether any two ads are written by the same or different author. The second is a technique that links specific ads to publicly available transaction information on Bitcoin. Using the cost of placing the ad and the time at which the ad was placed, we link a subset of ads to the Bitcoin transactions that paid for them. We then analyze those transactions to nd the set of ads that were paid for by the same Bitcoin wallet, i.e., those ads that are owned by the same person. As far as we are aware, this is the first work to explore this connection between paid ads and the Bitcoin blockchain, and attempt to link specific purchases to specific transactions on the Bitcoin blockchain.
In addition to reporting our results using our stylometry classifier on test sets of sex ads labeled by hard identifier, we apply both our tools to 4 weeks of scraped sex ads from Backpage, a well known advertising site that has faced multiple accusations of involvement with trafficking [10]. We assess the differences and similarities between the set of owners found using just hard identifiers, our stylometry model, the Bitcoin wallet, and finally all three combined. In summary, our contributions are as follows:
❖ We develop a stylometry classifier that distinguishes between sex ads posted by the same vs. different authors with 90% TPR and 1% FPR.
❖ We design a linking technique that takes advantage of leakages from the Bitcoin mempool, blockchain and sex ad site to link a subset of sex ads to Bitcoin public wallets and transactions.
❖ We propose two different methodologies that combine our classifier, our linking technique, and existing hard identifiers to group ads by owner.
❖ We evaluate our techniques on 4 weeks of scraped sex ads from Backpage, relying on the data automatically extracted using those two methodologies. We rebuild the price of each Backpage sex ad, and analyze the output of our two different methodologies.
We are working with two NGOs (Thorn and Global Emancipation Network) and one company (Marinus) who are all either currently using some subset of our tools and techniques, or are planning to work with us to incorporate our tools and techniques into their existing technology framework. Additionally, several law enforcement contacts have expressed a strong desire to deploy our tools in their own investigations once they become available.
The rest of this paper is organized as follows. Section 2 provides the necessary background for the rest of the paper. Section 3 outlines Backpage and Bitcoin, which we analyzed and used to evaluate our tools. Section 4 describes the methodology for building our stylometry classifier, covering ground truth labeling, the model we built, and validation results. Section 5 describes our linking technique. Section 6 describes our two proposed methodologies that combine our classifier, linking technique and existing hard identifiers to group ads by owner. Section 7 reports our findings when exploring the 4-weeks of scraped sex ads from Backpage, and Section 8 discusses limitations and future work. We conclude with reiteration of key contributions and findings.
Read more here.