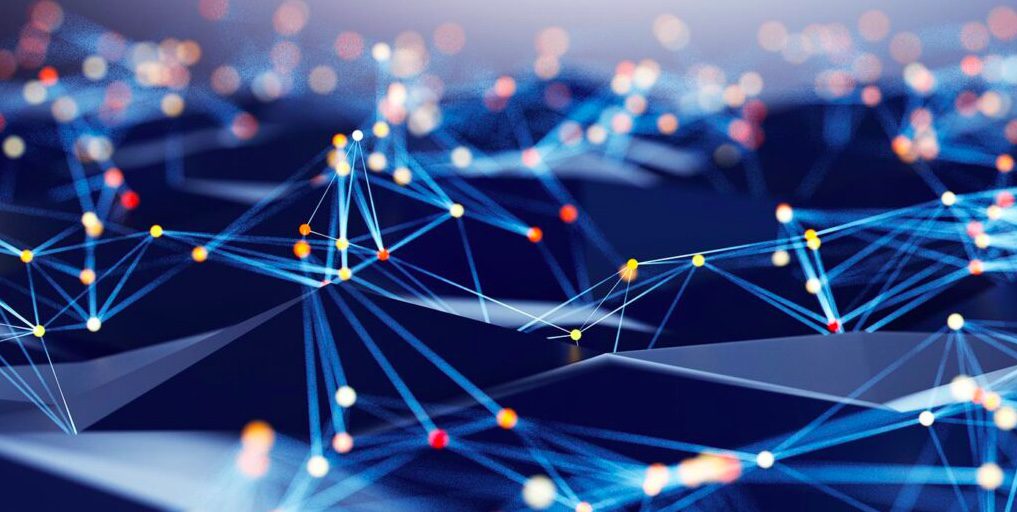
Building an Effective Data Ecosystem to Address Forced Labor in Global Supply Chains: Key Principles
Executive Summary
Modern slavery is on the rise with over 27 million people in situations of forced labor on any given day, with 86% estimated to be occurring in the private economy. This means forced labor is entrenched in global supply chains and, in part due to increased regulatory pressure from the US and Europe, has led to the development of a “supply chain data ecosystem” in which data flows between governments, policymakers, businesses and civil society organizations to meet demand for specific evidence of forced labor risks.
Despite an increasing amount of collected data about forced labor risks, the value and impact of that data is not being fully realized as the field continues to lack effective actions to prevent, mitigate and remediate actual cases of forced labor. This is in part because data is not being effectively shared between different actors in the supply chain data ecosystem.
Sharing data is not a goal in itself, but if purposefully done it can amplify the impact of anti-slavery policies, reduce the costs and duplication of data collection, and inform effective actions. It can help businesses better focus their resources and engagement efforts, governments target effective policies and enforcement, and civil society support greater impact.
This report, prepared by BSR as Secretariat of Tech Against Trafficking, presents:
- An analysis of the dynamics between actors in the data ecosystem that underpin efforts to address forced labor risks in global supply chains;
- A reflection on why data are or are not being shared; and
- principles that will enable data to be shared at scale, for a more effective data ecosystem.
A Fragmented Supply Chain Data Ecosystem
Our research confirms that the supply chain data ecosystem is fragmented, with significant data siloes between—and within—three universes: the corporate sector, civil society and the public sector.
The corporate sector is marked by contractually limited data collection and exchanges, and a general presumption against sharing data that could cause reputational damage to a company. Some sharing of risk data occurs between companies, but very little data flows across to or from the public sector or civil society.
- Many civil society organizations (including academia, journalists and non-governmental organizations) have embedded in their operating model the publication of data for the public good, and many actors from the public and private sector rely on these reports to inform their risk management or enforcement efforts. Yet siloes still exist, for example as a result of competitive pressures between NGOs, or academic publications behind paywalls.
- The public sector remains the most opaque, with limited visibility of what data is collected by frontline services such as law enforcement and labor inspectorates, and significant technical data management reducing access to this data from within and outside government. That said, intergovernmental organizations like the International Labor Organization and the International Organization for Migration support multi-lateral efforts to collect, aggregate and share relevant data on forced labor.
- The reasons for these silos include some technical limitations (e.g. limited technical or financial resources for digital data management) and legal limitations (e.g. data protection or security rules). But behavioral challenges remain the greatest barrier, and frequently come down to a lack of trust between organizations.
Common arguments such as the fear of legal action, anti-competitive behaviors or confidentiality obligations can often be overcome with the right expertise and motivation. Commercial concerns can also be a strong driver against sharing with third parties, for example deterring suppliers from sharing risk data with their customers, or NGOs and intergovernmental organizations from sharing data for fear or losing influence in a competitive funding market.
Opportunities to Overcome Barriers to Data Sharing
Nevertheless, examples of effective data sharing that overcome these challenges exist. Section 5 describes several examples shared by stakeholders consulted during this project: the RBA-Online, the Modern Slavery Intelligence Network, Stop the Traffik’s Traffik Analysis Hub, the ICT-ITC Sustainability Map collaboration, and the Crowdsourcing App for Responsible Production in Africa.
These examples surface common factors worth noting as we look to scale a more effective supply chain data ecosystem, including the creation of a sense of community bound by shared values and objectives, collective ownership and governance of the data-sharing process, the role of a trusted and expert intermediary and a user-friendly interface to upload or access shared data.
Tech Against Trafficking’s work with its Accelerator Program suggests existing and emerging technologies may also help overcome some of the barriers to data sharing identified in this report. Although these may also raise other human rights risks, such as risks to privacy, which should be carefully considered before deploying any of these methods.
Promising examples include the use of machine learning models to mass analyze data from public sources or structure unstructured data, or the development of federated data architectures or confidential computing to facilitate multilateral data flows between organizations. Machine learning may also enable privacy preserving techniques such as the automation of de-identification or the generation of synthetic data sets.
Toward A More Effective Supply Chain Data Ecosystem
Looking ahead, we envisage a more effective supply chain data ecosystem built around a federated or decentralized network of relationships where different actors collect and process data in a relatively harmonized manner, and are able to connect with each other directly or via data hubs. As this network of connections grows, and trust builds, more data will flow around the system, at greater scale, and for greater impact.
To achieve this future vision, this report identifies 7 areas for action by all actors involved. These are structured around three key principles to enable data sharing at scale for a more effective data ecosystem: the right data, the right resources, and the right behaviors.
To support these recommended actions, Tech Against Trafficking sees opportunities to collaborate with its members and partners identified through this research in three areas:
- Standardized and interoperable data, building on the ILO’s forced labor indicators and working in partnership with key standard-setting bodies and leading providers of supply chain risk data to align and build consensus around the most effective qualitative and quantitative datapoints to identify forced labor risk.
- Cost effective, accessible and scalable technologies for a federated ecosystem that enables organizations, including smaller and less technologically advanced actors, to share data while retaining control and privacy to the extent needed.
- Further dialogue between the public sector, civil society and the corporate sector on how governments can support a more effective supply chain data ecosystem