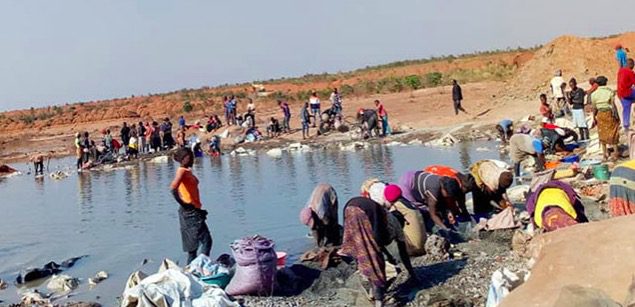
Addressing the social life cycle inventory analysis data gap: Insights from a case study of cobalt mining in the Democratic Republic of the Congo
Summary
Evaluating the human health and well-being effects of emerging technologies is essential. Yet, data to support rigorous evaluation of these effects through social life cycle assessment (S-LCA) are lacking, especially at local or regional rather than national scales. As a consequence, technologies and policies that use emerging technologies may drive inequality and detract from quality of life even if environmental life cycle assessments point to likely environmental benefits. Therefore, this Perspective describes our exploratory field work in cobalt mining communities in Lualaba Province, the Democratic Republic of Congo (DRC), to identify barriers to and opportunities for collecting better data for conducting S-LCA. Our recommendations apply to the S-LCA of cobalt mining and other systems and, overall, enable more holistic evaluations of emerging technologies’ effects on social well-being that are insufficiently robust for use in policy.
Introduction
Environmental life cycle analysis (ELCA) is a mature, widely used tool that has been formalized by the International Standards Organization (ISO)1,2 to systematically evaluate the environmental effects of products, processes, and systems.1In an ELCA, material and energy flows for a product, process, or system are cataloged at each stage of a supply chain, including raw material extraction and processing, transportation and distribution to a consumer, use, and end-of-life. There are four phases of anELCA (Figure 1): 1) definition of goal and scope, including, for example, specifying the system boundary; 2) life cycle inventory analysis, in which system inputs and outputs are identified and quantified using empirical data (e.g., electricity consumption)or engineering models; 3) life cycle impact assessment, in which system-level indicators are evaluated (e.g., global warming potential), using data collected from the prior phase; and 4) interpretation, in which analysts use Phase 3 results to identify the most significant life cycle impacts and communicate strategies for addressing them to relevant stakeholders.1,This four-stage process permits a holistic assessment of a system’s associated environmental impacts, including GHG emissions, water consumption, and air pollution.Within the transportation sector, ELCA has been used to examine the environmental impacts of EVs 4,5 and the cobalt-containing lithium-ion batteries that power them,6,7 as well as other potentially low-GHG-emitting transportation options including biofuels,8 fuel cell vehicles,9,10 and vehicles using natural gas.11
The environmental effects of these options are then compared against those of a well-characterized baseline, such as conventional gasoline- or diesel-fueled transportation, to understand whether these systems offer advantages (e.g., lower GHG emissions per mile) or how they might be tailored to enhance environmental benefits.12As such, ELCA provides an evidence base for policymakers, corporations, and consumers to incentivize, develop, or purchase vehicle technologies that reduce GHG emissions and minimize broader environmental effects (e.g., on water and air quality). Accordingly, findings from ELCA have been used to develop policies and regulatory standards in the US13and Europe.14 ELCA does not, however,consider how a technology impacts human health and wellbeing beyond environmental quality and resource use.
For Example, ELCA does not consider safe living conditions or fair wages. Social LCA (S-LCA) has the potential to complement and expand upon the information generated by ELCA. Analogous to ELCA, S-LCA aims to assess the impacts of a product, process, or system on the health and well-being of humans and their surrounding communities .3, 15, 16S-LCA and ELCA differ, though, in their Phase 2 and 3 aims, methods, and indicators. For example, Phase 2 (inventory analysis) of ELCA involves characterization of material and energy flows through direct measurement or estimation with engineering models, whereas information about the health and well-being of stakeholders in a supply chain is collected for S-LCA. Data from the inventory analysis are then used to create aggregate indicators (Phase 3). sources and data collection methods for Phases 2 and 3 of ELCA have been standardized by ISO,1,2allowing for comparison of results across studies and systems. In contrast, indicators for S-LCA have yet to be formalized,17–19 making it difficult to determine the relative impacts of different processes and thereby limiting the tool’s utility. Some groups, such as the Roundtable Of Product Social Metrics, have developed standardized indicators, but methodological differences and data unavailability, particularly at high resolution, remain as challenges.16 There are multiple, and sometimes divergent, frameworks and methodologies applied to S-LCA.16–20 These differ in terms of the overall goal (e.g., identifying hotspots in a supply chain versus comparing the social effects of multiple product options),social impacts measured, type of data included (e.g., qualitative or quantitative), method of data collection (e.g., from a database for interviews), and techniques to aggregate data into comparable indicators.
Further, some frameworks guide the evaluation of social impacts for a single corporation, which has limited use for guiding policy development.21,22In contrast, frameworks that develop indicators using a ‘‘functional unit,’’ such as impacts per mile traveled or per product produced,23,24 are easier to compare across studies and often more actionable for governmental decision makers.For S-LCA to reach its potential in the policy arena as a preferred decision-making tool as ELCA has done, the data that are the basis for S-LCA must be robust, efficient, trans-parent, and sufficiently specific to the regions that are relevant to the supply chain. The lack of guidance on best practices for conducting S-LCA, including a dearth of information about which data collection methods should be used, among which populations, and at which timepoints, has ultimately made it difficult to collect high-quality data that are comparable across settings,time, and studies. Furthermore, interviews and focus group discussions—which are often promoted in S-LCA guidelines—are resource intensive, yet there is little discussion of opportunities to use other data sources. Although it is well recognized that data for S-LCA are scarce,15,17–19 most research to date has Figure 1. ELCA and S-LCA framework.
The four phases of life cycle assessment with illustrative examples of how ELCA and S-LCA are similar and different. Both types of life cycle analyses are iterative processes and there are interactions between phases.focused on the development of new frameworks for conceptualizing S-LCA, rather than identifying available data sources or methods for collecting data necessary for conducting S-LCA.19 For example, Tokede and Traverso 16 review the different theories, methodologies, and indicators used in the recent S-LCA literature, but the scope of their review did not include a discussion of specific techniques to improve life cycle inventory analysis. Correspondingly, unlike ELCA, S-LCA remains mostly theoretical and has not yet become a widely accepted methodology or evaluation framework.25To expand our understanding of the barriers and opportunities to improving data collection in S-LCA life cycle inventory analysis(Phase 2), we developed a social-natural science collaboration to identify generalizable methods, using cobalt mining in the Democratic Republic of the Congo (DRC) as a case study.
The exploratory fieldwork for our brief case study, which afforded greater insights into our objectives than we could obtain from literature review alone, was carried out among cobalt mining communities in Lualaba Province, the DRC. We employed key informant interviews and focus group discussions in this work.Rather than producing a comprehensive dataset for S-LCA, we sought to identify the most salient human health and well-being effects of mining in the Province as well as existing and potential data sources for use in S-LCA among the two stakeholder groups most likely to be adversely affected by cobalt extraction:miners (classified as ‘‘workers’’ using terminology developed by the United Nations Environment Program) and local communities. Based on our findings, we suggest a number of quantitative and qualitative techniques that draw on social science methodologies, in addition to emerging data sources, including high-frequency, high-resolution satellite imagery, that have the potential for use in Phase 2 of S-LCA. Ultimately, the ability to incorporate efficient, low-cost methods that draw on interdisciplinary expertise and capture local-scale effects will expand the utility and comparability of S-LCA for policy.
Read full report here.